Signal Processing in Healthcare
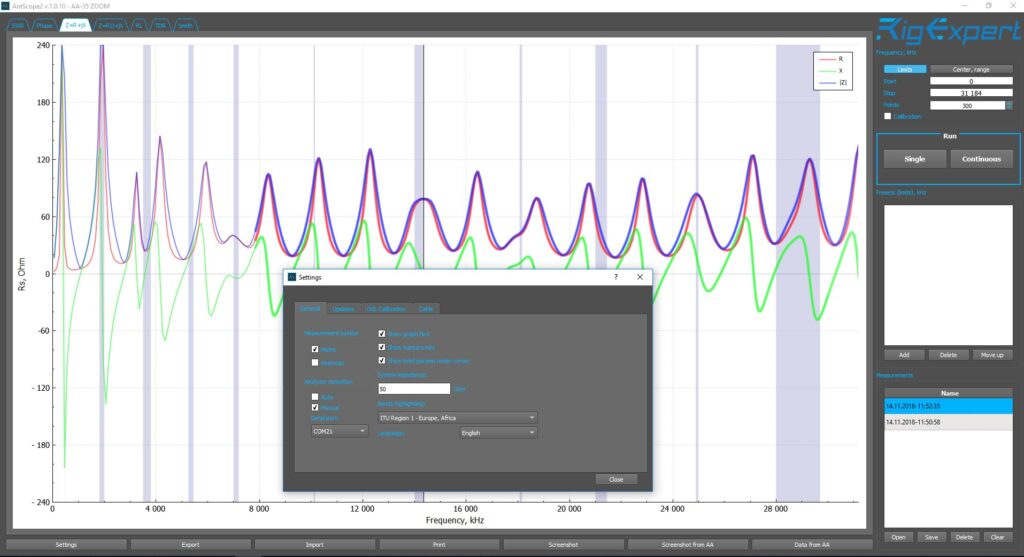
Understanding Signal Processing in Healthcare
Signal processing in healthcare refers to the application of mathematical algorithms and techniques to analyze, interpret, and manipulate physiological signals, medical images, and other healthcare data. These signals may include electrocardiograms (ECG), electroencephalograms (EEG), medical images from modalities like MRI and CT scans, genetic data, and more.
The goal of signal processing in healthcare is to extract meaningful information from these signals to aid in diagnosis, monitoring, treatment, and research. These techniques are used to enhance the quality of medical data, reduce noise and artifacts, extract relevant features, detect abnormalities, classify patterns, and make predictions.
In practical terms, signal processing in healthcare involves a wide range of applications, including remote health monitoring using wearable devices, medical imaging analysis for disease diagnosis, analysis of genetic data for personalized medicine, and more. It plays a crucial role in advancing medical research, improving patient care, and ultimately enhancing health outcomes.
Application of Signal Processing in Healthcare
- Signal processing techniques are employed in wearable devices and medical sensors to monitor vital signs such as heart rate, blood pressure, and respiratory rate. These devices collect continuous streams of data, which are processed to detect anomalies and patterns indicative of health status changes.
- It plays a pivotal role in medical imaging modalities like MRI, CT scans, and ultrasound. It involves enhancing image quality, reducing noise, and extracting relevant features for accurate diagnosis and treatment planning.
- Signals obtained from biological sources, such as brain activity (EEG), heart activity (ECG), and muscle contractions (EMG), are analyzed using signal processing techniques to extract diagnostic information. This includes detecting abnormalities, quantifying physiological parameters, and identifying patterns associated with specific medical conditions.
- With the advancement of telemedicine, signal processing facilitates remote patient monitoring. Through the analysis of transmitted signals, healthcare professionals can remotely assess patients’ health status, provide timely interventions, and make informed decisions without the need for physical presence.
- It enables the customization of healthcare interventions based on individual patient characteristics and responses. By analyzing patient-specific data, such as genetic information, medical history, and real-time physiological signals, personalized treatment plans can be developed to optimize outcomes and minimize adverse effects.
- These techniques are continually evolving to meet the growing demands of healthcare. Researchers are exploring innovative algorithms and technologies to improve signal quality, increase diagnostic accuracy, and enhance patient care.
See Also: Health Technology: Wearables and Smart Devices In Healthcare Today
Signal Processing Techniques for Medical Imaging
- Filtering techniques such as linear filtering, median filtering, and Gaussian smoothing are used to reduce noise in medical images while preserving important features. Filtering helps improve image quality by enhancing contrast and reducing artifacts.
- Image enhancement techniques aim to improve the visual quality of medical images by increasing their contrast, sharpness, and overall clarity. Histogram equalization, contrast stretching, and edge enhancement are examples of image enhancement techniques commonly used in medical imaging.
- In modalities like computed tomography (CT) and magnetic resonance imaging (MRI), signal processing techniques are employed for image reconstruction from raw data acquired during scanning. Reconstruction algorithms such as filtered, back projection, and iterative reconstruction improve image quality and reduce artifacts.
- Signal techniques are used to extract relevant features from medical images for quantitative analysis and diagnosis. Feature extraction methods, including texture analysis, edge detection, and shape analysis, help identify regions of interest and characterize tissue properties.
- Image registration techniques align multiple images acquired from different modalities or time points to facilitate comparison and analysis. Registration methods involve spatial transformation and optimization algorithms to align images based on anatomical landmarks or intensity patterns.
- Segmentation techniques partition medical images into anatomically meaningful regions, or structures, for quantitative analysis and visualization. Segmentation algorithms, such as thresholding, region growth, and active contours, assist in delineating organs, tumors, and other abnormalities.
- Segmentation techniques partition medical images into anatomically meaningful regions, or structures for quantitative analysis and visualization. Segmentation algorithms, such as thresholding, region growth, and active contours, assist in delineating organs, tumors, and other abnormalities.
- Image fusion techniques integrate information from multiple imaging modalities or imaging sequences to create composite images with enhanced diagnostic value. Fusion methods combine complementary information from different modalities, such as MRI and positron emission tomography (PET), to improve disease detection and characterization.
- Image compression techniques reduce the storage and transmission requirements of medical images while preserving diagnostic information. Compression algorithms, such as JPEG and wavelet-based compression, achieve high compression ratios without significant loss of image quality.
- Signal processing techniques are employed to correct artifacts resulting from imperfections in imaging equipment or patient motion. Artifact correction methods, including motion correction, gradient distortion correction, and metal artifact reduction, enhance image quality and accuracy for reliable diagnosis.
- Deep learning approaches, particularly convolutional neural networks (CNNs), are increasingly used in medical imaging for tasks such as image classification, segmentation, and reconstruction. Deep learning models trained on large datasets can automate image analysis tasks and improve diagnostic accuracy.
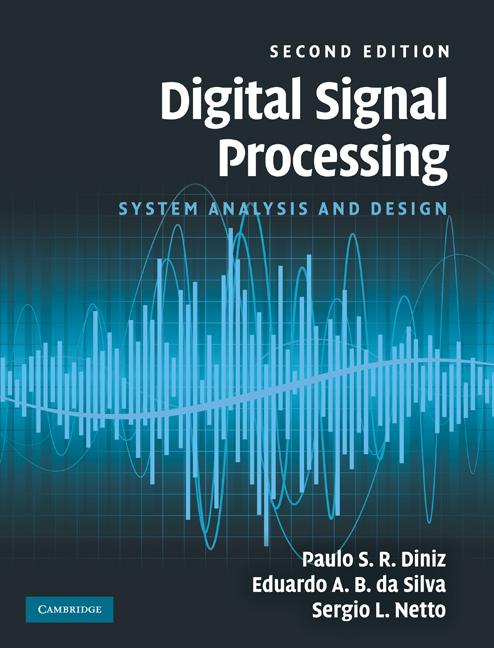
Wearable Devices and Signal Processing for Remote Health Monitoring
- Wearable devices incorporate sensors to measure physiological parameters such as heart rate, blood pressure, temperature, oxygen saturation, and activity levels. These sensors may include photoplethysmography (PPG) sensors, accelerometers, gyroscopes, electrocardiogram (ECG) sensors, and more.
- Wearable devices continuously collect raw sensor data from individuals throughout the day. This data includes signals representing physiological parameters as well as contextual information such as activity levels, sleep patterns, and environmental factors.
- Signal processing algorithms are applied to the raw sensor data to extract meaningful information and derive health insights. These algorithms include noise filtering, feature extraction, pattern recognition, and machine learning techniques tailored to specific health monitoring tasks.
- Signal processing techniques are used to extract relevant features from the raw sensor data, such as heart rate variability, respiratory rate, and activity patterns. These features provide valuable insights into individuals’ health status and physiological functioning.
- Signal processing algorithms analyze extracted features to detect anomalies or deviations from normal patterns. For example, abnormal heart rate rhythms, fluctuations in blood pressure, or sudden changes in activity levels may indicate health problems or emergencies, triggering alerts for further assessment.
- Wearable devices often integrate data from multiple sensors to provide a comprehensive view of individuals’ health. Signal processing techniques are used to fuse data from different sensors, compensating for sensor limitations and improving the accuracy of health monitoring.
- Signal processing enables real-time analysis of sensor data, allowing wearable devices to provide immediate feedback and alerts to users and healthcare providers. Real-time monitoring enables timely intervention in case of health emergencies or abnormalities.
- Wearable devices store historical sensor data, allowing for long-term trend analysis and monitoring of individuals’ health over time. Signal processing techniques are used to analyze trends, detect gradual changes in health parameters, and identify risk factors for chronic conditions.
- Wearable devices transmit processed health data to smartphones, tablets, or cloud-based platforms for further analysis and storage. Signal processing techniques may continue to be applied during data transmission to reduce bandwidth requirements and ensure data integrity.
- Signal processing algorithms analyze individuals’ health data to generate personalized insights and recommendations for health management. These insights may include recommendations for lifestyle modifications, medication adherence, or preventive healthcare measures based on individual health profiles.
Signal Processing in Biomedical Signal Analysis
Signal processing plays a critical role in the analysis of biomedical signals, which are recordings of physiological activities within the human body. These signals, such as electrocardiograms (ECG), electroencephalograms (EEG), electromyograms (EMG), and many others, provide valuable insights into the functioning of various organs and systems. Here’s how signal processing is used in biomedical signal analysis:
- Biomedical signals are often contaminated with noise and artifacts due to various sources such as electrical interference, motion artifacts, and biological variability. Signal processing techniques, including filtering, noise reduction, baseline correction, and artifact removal, are applied to preprocess the signals and improve their quality for subsequent analysis.
- Signal processing methods are used to extract relevant features from biomedical signals that capture important characteristics related to physiological events or abnormalities. Feature extraction techniques, such as time-domain analysis, frequency-domain analysis, and time-frequency analysis, quantify signal properties such as amplitude, frequency, duration, and variability.
- Biomedical signals contain patterns and structures that are indicative of specific physiological conditions, diseases, or events. Signal processing algorithms, including pattern recognition, classification, and machine learning techniques, are applied to detect, classify, and interpret patterns in biomedical signals for diagnostic purposes. For example, ECG signal processing can identify cardiac arrhythmias, while EEG signal processing can detect epileptic seizures.
- Biomedical signal analysis often involves the integration of multiple signals acquired from different modalities or sensors to improve diagnostic accuracy and reliability. Signal fusion techniques combine information from diverse sources, such as ECG, EEG, and EMG, to provide a comprehensive assessment of physiological processes and pathological conditions.
- Biomedical signals may exhibit temporal and spatial variations that convey important physiological information. Signal processing techniques enable the analysis of temporal dynamics, such as heart rate variability in ECG signals or event-related potentials in EEG signals. Spatial analysis techniques, such as spatial filtering and source localization, are used to localize and characterize neural activity in the brain based on EEG or functional magnetic resonance imaging (fMRI) data.
- Biomedical signals often comprise multiple underlying components or sources that contribute to the observed signal. Signal decomposition techniques, such as independent component analysis (ICA) and wavelet transform, decompose complex signals into their constituent components, facilitating the separation and analysis of individual physiological phenomena or artifacts.
- Biomedical signals exhibit dynamic behaviors and interactions within physiological systems. Signal processing methods enable the construction of dynamic models, such as mathematical models, system identification models, and dynamic network models, to characterize the underlying dynamics, predict future behavior, and simulate physiological responses to external stimuli or interventions.
- Biomedical signal analysis provides valuable information for clinical decision-making in healthcare. Signal processing techniques aid in the interpretation of biomedical signals, assisting healthcare professionals in diagnosing diseases, monitoring treatment effectiveness, predicting prognosis, and guiding therapeutic interventions.
Challenges and Future Directions in Signal Processing for Healthcare
- As healthcare data continues to grow in volume and complexity, signal processing algorithms must be able to efficiently handle large-scale datasets generated from wearable devices, medical imaging modalities, and electronic health records. Future directions involve developing scalable and real-time processing techniques to extract actionable insights from big data while ensuring privacy and security.
- Integrating heterogeneous healthcare data sources and systems poses challenges in interoperability and data exchange. Future directions include developing standardized formats, protocols, and interfaces to enable seamless integration of data from diverse sources, facilitating holistic patient care and data-driven decision-making.
- Tailoring healthcare interventions to individual patient characteristics and preferences requires personalized signal-processing techniques. Future directions involve leveraging machine learning and artificial intelligence to develop personalized models and algorithms that adapt to individual patient needs, enabling precision medicine and personalized healthcare delivery.
- Real-time signal processing for continuous health monitoring and decision support is essential for early detection of health abnormalities and timely intervention. Future directions involve developing low-latency algorithms and edge computing solutions to enable real-time processing of biomedical signals, facilitating rapid clinical decision-making and interventions.
- Signal processing algorithms must be robust to noise, artifacts, and variations in data quality to ensure reliable and accurate results. Future directions include incorporating robust optimization techniques, adaptive algorithms, and uncertainty quantification methods to improve the reliability and robustness of signal processing in healthcare applications.
- Ethical and regulatory challenges related to data privacy, consent, transparency, and accountability must be addressed to ensure the responsible use of healthcare data and signal processing techniques. Future directions involve developing ethical frameworks, governance mechanisms, and regulatory standards to guide the ethical conduct and responsible deployment of signal processing technologies in healthcare.
- Integrating information from multiple modalities, such as biomedical signals, medical images, genomic data, and clinical records, offers valuable insights into patient health and disease. Future directions involve developing multimodal data fusion techniques that combine complementary information from diverse sources, enabling comprehensive patient profiling and personalized healthcare management.
- Analyzing longitudinal healthcare data over extended periods enables the identification of disease trajectories, predictive modeling of health outcomes, and early intervention strategies. Future directions involve developing longitudinal analysis techniques, predictive modeling algorithms, and decision support systems that leverage historical data to anticipate future health risks and optimize healthcare delivery.
Ethical Considerations in Signal Processing Applications for Health
- Signal processing algorithms often require access to sensitive health data, including medical records, genetic information, and physiological signals. Ensuring data privacy and confidentiality is essential to protect individuals’ rights and prevent unauthorized access or misuse of their personal health information.
- Individuals should have the right to provide informed consent before their health data is collected, processed, or shared for signal processing applications. Informed consent involves providing clear and understandable information about the purpose, risks, and potential benefits of data processing, and allowing individuals to make voluntary decisions about their participation.
- Signal processing algorithms should be transparent and accountable, with clear documentation of their design, implementation, and performance metrics. Transparency enables individuals to understand how their health data is being used and facilitates accountability for algorithmic decisions and outcomes.
- Signal processing algorithms should be designed and evaluated to mitigate biases and ensure fairness in healthcare decision-making. Biases in data collection, algorithm design, or training data can lead to unfair treatment or disparities in healthcare outcomes, particularly for marginalized or underrepresented populations.
- Signal processing systems should implement robust security measures to protect healthcare data from unauthorized access, alteration, or corruption. Ensuring data security and integrity is essential to maintain trust in signal processing technologies and safeguard individuals’ health information against cyber threats and breaches.
- Signal processing applications should respect individuals’ autonomy and empower them to make informed decisions about their health. Providing individuals with access to their health data, tools for self-monitoring, and opportunities for shared decision-making promotes patient autonomy and engagement in healthcare management.
- Signal processing researchers and practitioners should adhere to ethical principles and guidelines throughout the research and development process. Responsible research and innovation involve considering the potential societal impacts, risks, and ethical implications of signal processing technologies and integrating ethical considerations into the design, deployment, and evaluation of healthcare solutions.
- Signal processing applications for health should comply with relevant laws, regulations, and ethical standards governing data protection, healthcare privacy, and medical device safety. Compliance with regulatory requirements helps ensure the legal and ethical use of signal processing technologies and promotes public trust in healthcare systems.
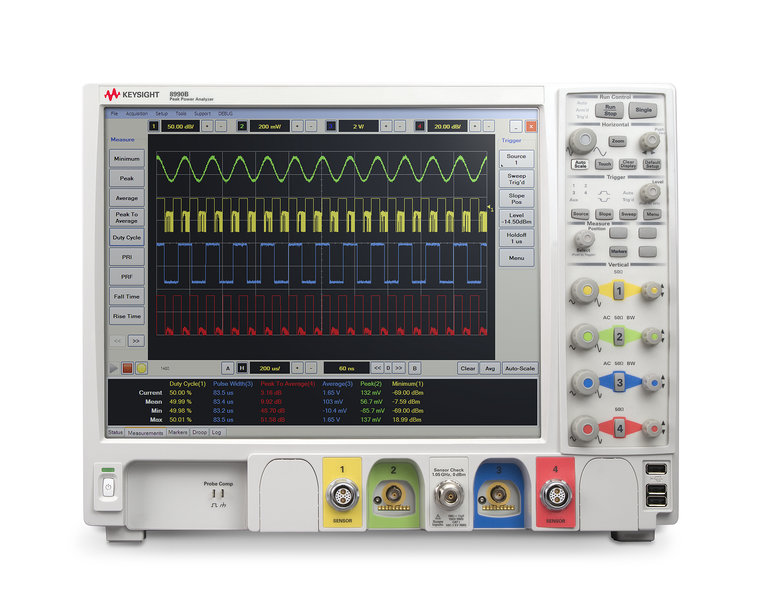
Conclusion
In conclusion, signal processing plays a pivotal role in transforming healthcare delivery by enabling the analysis, interpretation, and utilization of diverse biomedical data. From wearable devices for remote health monitoring to advanced imaging techniques for disease diagnosis, signal processing algorithms extract valuable insights from complex healthcare data, improving patient outcomes and enhancing clinical decision-making.
However, the widespread adoption of signal processing technologies in healthcare comes with ethical considerations that must be carefully addressed. Ensuring data privacy, informed consent, transparency, fairness, and accountability is essential to uphold individuals’ rights, autonomy, and trust in healthcare systems.
Looking ahead, the future of signal processing in healthcare holds immense promise. Advancements in big data handling, personalized medicine, real-time monitoring, and responsible innovation will further enhance the capabilities of signal processing technologies to revolutionize healthcare delivery. By embracing ethical principles and regulatory compliance, signal processing applications can contribute to ethical, equitable, and patient-centered healthcare practices, ultimately improving health outcomes and quality of life for individuals worldwide.
Frequently Asked Questions (FAQs)
What is signal processing in healthcare?
Signal processing in healthcare involves the analysis, interpretation, and manipulation of physiological signals, medical images, and other healthcare data to extract meaningful information for diagnosis, monitoring, and treatment.
How is signal processing used in health monitoring?
Signal processing techniques are used in wearable devices to monitor vital signs such as heart rate, blood pressure, and activity levels. These devices collect data from sensors and apply signal processing algorithms to analyze and interpret the data for remote health monitoring.
What are some applications of signal processing in medical imaging?
Signal processing techniques are used in medical imaging modalities such as MRI, CT scans, and ultrasound to enhance image quality, reduce noise, and extract relevant features for accurate diagnosis and treatment planning.
What are the challenges in signal processing for healthcare?
Challenges in signal processing for healthcare include handling big data, ensuring interoperability and integration of diverse data sources, addressing ethical and regulatory considerations, and developing robust and reliable algorithms for real-time monitoring and decision support.
How can signal processing contribute to personalized medicine?
Signal processing techniques enable the analysis of individual patient data to customize healthcare interventions based on specific patient characteristics and responses. This personalized approach, known as precision medicine, aims to optimize treatment outcomes and minimize adverse effects.